Cancellation Rates in SaaS Business Models
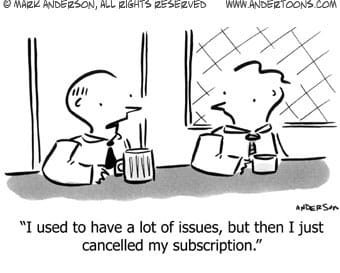
Why cancellation rate is so important
As a preamble to the metrics, it’s useful to know what you’re measuring and why it’s vital.
[Cancellation rate] = [product utility] + [service quality] + [acceptable price]
I put in these particular elements because I did a study of the reasons people cancel at WP Engine, and these are the main reasons for cancellation. We log every cancellation – spending time running after folks to wring out the cause — so we can deduce exactly what we can do to prevent it in future. (Of course you should do this too and get your own data.)
These three factors are, of course, critical to a healthy, growing startup, and yet individually they’re impossible to measure as precisely and easily as cancellation rate. (I’ve never seen a graph of “usefulness of the product.”) So although it’s a single number combining several factors, and we know that averaging can obfuscate, I think cancellation rate is a good overall measure of how well the company is servicing its customers, and with tools like our detailed log you can still break apart the single number into concrete, actionable influences.
Beyond the analytical breakdown, I have an emotional attachment to this number, because whenever someone cancels I think about what had to happen to get them to this point, and it kills me. Of course people cancel only after they’re already a customer, which means they’ve already gotten through the barriers preventing them from buying: finding your website, not bouncing off the home page, understanding what you offer, deciding it’s something they want, researching the competition, signing up, configuring settings, entering a credit card number, rolling through tech support, and maybe even announcing to some Facebook “friends” that they just found something cool.
Barely anyone on Earth will ever power through this gauntlet. I turn myself inside out just to get a thousand people to bounce off the home page, praying that one makes it through to the end, like a frog laying ten thousand eggs hoping three survive long enough to do the same.
And then, after all that… they cancel! Son of a bitch! I have to know why and I have to do something about it!
So we’re going to measure this bastard, and we’re going to compute another useful thing from it, but it turns out to be harder than it first appears.
The many kinds of cancellation rate
A “rate” is a ratio of “something divided by time,” and it’s unclear what the something is and over how much time.
There are many kinds of so-called “cancellation rate,” each subtly but critically different.
All of the following definitions are meaningful, but each measures something different. It’s useful to describe each so you can decide which (or several!) make sense in your case:
- Percentage of current customers who canceled in a given day/week/month. If this spikes, something just changed in how you’re behaving across the board. We had a spike in this metric in February at WP Engine when our Internet provider themselves had a datacenter-wide catastrophe which brought us down for twelve hours; of course not all spikes will have such obvious causes. We’ve also rolled out new initiatives designed to reduce this rate, and for the most part we’ve been successful. When selecting the duration component, it has to be long enough to get a stable number, but not so long that sharp changes in reality take weeks to register on the chart; I recommend using smallest possible time period without seeing the metric hit “0%.”
- Percentage of new customers in a given month which end up canceling at any later date. This is often called “cohort analysis,” where the new customers in January are tracked together as one cohort, new customers from February as another, etc.. The idea is to compare the behavior of each cohort against subsequent cohorts, but comparing similar periods in those customers’ lifespans. For example, how many who started in Jan cancelled in Feb compared to how many who started in Feb cancelled in Mar, and so on. This determines not whether your service is improvingacross the board, but whether new customers are getting a better newexperience. This is especially useful if — like most companies — you have a higher cancellation rate with new customers than with old ones, and you (think you) have taken steps to improve that situation, and want to measure that progress.
- Absolute number of cancellations per week. This metric is supposed to increase over time at a growing SaaS company simply because there are more and more customers available to cancel. Still, you should also be getting better at preventing cancellations, or at least certain types of cancellations, such as crappy tech support or lacking a feature. Our cancellation log implicitly represents this metric because we review it weekly to look for trends. It’s not something we feel is also useful to graph because changes in the number aren’t necessarily actionable.
- Percentage of all customers who’ve cancelled over the lifetime of the company to-date. This is another way of measuring an “in/out” ratio — plotting relative number of new customers arriving versus customers exiting. For a company laser-focussed on accelerating the number of active users, it might be actually worth having high cancellation rates if it meant an even higher acquisition rate. This metric measures this relative change, so long it’s decreasing (or not increasing), you might be happy even if you’re not watching or optimizing for the cancellation rate in isolation. For a quality-service company like WP Engine a high cancellation rate is a sign of terminal cancer even if acquisition rates are also increasing, so this metric isn’t useful to us. It’s also not particularly useful when, again like WP Engine, the monthly cancellation rate is nice and low while new customer acquisition is healthy, because in this case — by definition — this metric diminishes asymptotically. Whenever you know for certain what a metric will do, it’s not useful or actionable to measure it!
- Cancellations as deactivations. For paid services like WP Engine, a “cancellation” is literally “the customer called to cancel, or clicked the ‘stop charging my card’ button.” For many consumer-Internet companies, most of your users aren’t paying, and therefore almost none will bother to take a cancel “action” even if they’re effectively cancelled. Rather, a lack of activity signifies an effective cancellation. In this case, you need to have a clear definition of an “active user” (e.g. “has logged in at least once in the past week”) and consider the user “cancelled” if they were active before but now are not.
- Revisionist history. What happens when a customer cancels but then returns? This is relatively rare in a company like WP Engine but is common for those consumer-Internet companies where cancellation is identical to passive deactivation, because a user might be reinvigorated by a newsletter or a tweet. In that case, you can decide that user didn’t cancel after all and update historical data in your charts. That’s perfectly fine, just as it’s perfectly fine that a customer today might become a cancellation tomorrow but that’s not yet in the chart either.
Of all these techniques, the one that is perhaps most important and useful for us at WP Engine — and likely you too — is cancellation rate by age. I call this a “continuous version of cohort analysis.”
You take the number of cancellations in a given time period (we use months), broken out by age (e.g. younger than 30 days old versus older), and then compute the “cancellation rate” as a percentage of all customers in that age group who cancelled. Like many companies, we’ve found that people who cancel soon after sign-up do so for very different reasons than those who cancel after a year, and we care about those two groups differently, and we act on them differently.
For example, quick cancels are often due to situations things like “I decided I didn’t want a blog after all” or even “My son used my credit card to set up my website but I’m not going to pay for hosting.” We probably can’t affect these circumstances much, or at least it’s not worth our time to try. So we’ll never get the short-term cancellation rate lower than a certain number — a number we can actually compute by marking these in the cancellation log and totting them up separately.
This is useful, not only because it sets a sensible target “floor” for our activities that do reduce short-term cancellation, but because it calibrates our expectations on how much front-end sign-ups we need to achieve our growth targets. It’s an “automatic drag” that we can just factor in to our projections.
On the other end of the time spectrum, every time we lose a long-term customer — which is thankfully almost never, knock wood — it’s cause for us to sit up and do a post-mortem. That rate had better be very close to 0%. If it ever pops it would be a “tools-down, everyone get on that right now” sort of problem.
I do worry about this happening as we grow, as should any company. It’s common knowledge that expanding companies have a hard time maintaining the level of service which earned them that growth. You sign up customers faster than your ability to hire quality people, so either existing people are stretched thin or you make the more fatal error of lowering standards to fill chairs. It’s harder to train people and keep your culture going, and those founders who were brilliant at seeking market-fit and constructing the foundation of a startup might be ill-suited for scaling that organization. It appears some of our WP Engine competitors are experiencing exactly this, right now. An increase in our long-term cancellation rate will be our first warning sign that we’re not handling the growth properly, so we watch it like a hawk.
LTV, my way
The other useful thing we do with cancellation rate is to compute LTV (customer LifeTime Value), but I don’t use the simplistic technique espoused by many others.
“LTV” means “the total revenue you’ll get from a customer over its lifetime.” For a simple subscription business model the formula is easy to write but hard to compute:
[LTV] = [monthly revenue] × [number of months in lifetime]
The hard part is “number of months,” because of course you don’t know how many months you’ll keep a customer until that customer leaves, and hopefully most haven’t left yet. Worse, if you’re like WP Engine you haven’t been around long enough to know how long most customers will stick around. For example, WP Engine is 15 months old and we’ve retained 95% of our customers, but thelongest anyone has stayed is by definition 15 months, and because we’re growing, the average is probably around 6! But how long will all these folks stick around if we keep at it for another five or ten years?
P.S. Who cares about LTV? It’s the main way to determine whether your company is profitable and how much money you can (should?) spend on marketing, but that subject is covered well elsewhere so I’ll skip this and get back to geeking out over math.
It turns out you can compute the elusive “expected number of months” from your cancellation rate, even if you only have a few months of data to go on.
The typical formula is derived like so:
- Let p be the percentage of current customers who cancel in a given month. For example, if in March you had 200 paying customers and 10 cancelled,p = 0.05.
- In any month of N customers, Np will cancel, leaving N(1-p).
- Assuming you didn’t add any new customers, you would bleed customers according to that formula, every month. The customers remaining would be N(1-p) after the first month, then N(1-p)(1-p) after the second, then N(1-p)(1-p)(1-p) after the third, and so forth.
- In each month we get $R revenue per customer, so that means the first month we get $RN, then $RN(1-p), then $RN(1-p)(1-p), and so forth.
- To compute the “lifetime” amount of revenue these customers provide, you add up this infinite series, so: $RN + $RN(1-p) + $RN(1-p)(1-p) + $RN(1-p)(1-p)(1-p) + …
- Factor out the $RN, so you get: $RN × [1 + (1-p) + (1-p)(1-p) + … ]
- That bracketed infinite series can be rewritten as just 1/p.
- So total expected revenue is $RN/p.
- And the average expected revenue per customer is $R/p.
- And the average number of months per customer is 1/p.
So with our example of a 5% monthly cancellation rate, p = 0.05 so expected months is 20. If it’s a base-level WP Engine customer, that’s 20 months at $50 per month, so $1000 total LTV.
It’s nice when the math turns out simple, but that doesn’t mean it’s right.
It is right if your cancellation rate is indeed p every month, for every customer. But I just got through explaining that this isn’t at all the case. Newer customers tend to have high cancellation rates; older ones much smaller. In fact it’s not unusual for there to be a 10x difference between the short-term and long-term rates.
Therefore, I suggest a hybrid approach: First compute expected survivors over the short-term cancellation period, then use the “infinite sum” technique for the long-term customers.
Running an example will make this clear. Using the “cancellation rate by age” metric, suppose for the first 3 months of life your customers’ cancellation rate is 15%/mo, but after that the rate is 3%/mo.
So you retain 85% of your customers after the first month, 85% of those after the second, etc., for three months, for a retention after three months of (0.85)3 = 0.61.
I then completely ignore the revenue received by those 39% of customers who stuck around for only a few months. Sure they gave us a little money, but surely it’s negated by the time-cost of messing with them over tech support and processing cancellations and refunds. I want LTV to be a conservative metric, so I ignore this revenue.
Now, with 61% of my original customers remaining, it makes sense to use the formula above to predict they’ll stick around for another 1/0.03 = 33 months, and since they’ve already made it through 3 months, that’s a grand total of 36 months.
So, for a brand new customer, there’s a 61% chance they’ll deliver 36 months’ of revenue, and 39% chance I get nothing (significant), for an expected 0.61 × 36 = 22 months on average.
To demonstrate why this method, while more tedious, is superior than the simplistic one, observe that if you ignore age groups, this same company would appear to have a 6% cancellation rate (which conceals the interesting customer behavior) and by the usual formula would have expected months of 1/0.06 = 16 months, which is incorrect by almost 40%. That’s a lot of error!
For those of you who like formulas, we can compile the example down to variables:
- r = short-term cancellation rate (e.g. 0.15)
- p = long-term cancellation rate (e.g. 0.03)
- s = number of months in the “short-term” age group (e.g. 3)
- (1-r)^s × (s + 1/p) = expected months
Yes, this is how I compute LTV at WP Engine, except worse! Because we’ve decided there’s actually three distinct age-groups, plus we treat coupon-based customers separately because have a significantly higher cancellation rate, often for reasons that have nothing to do with our behavior.
As a final note on LTV, I personally don’t care much about lifetime revenue, and prefer to compute lifetime operational profit, meaning the net revenue after taking out known costs of service. In the case of WP Engine, we knock off 2% for credit card fees and a certain amount for hosting and bandwidth costs.
Once you get down to that, you can easily answer questions like “how much money can we spend to acquire a customer.” For example, if LTV (net) is $500, it’s a pretty easy decision to spend $50 or even $150 to acquire a customer. That might mean AdWords, tradeshows, give-aways, coupons, affiliate programs, or anything! Extremely useful for confidently measuring marketing campaigns against profitable customer growth.
I guess that last sentence sounded like abstract business-speak drivel. But it’s true, and getting a solid handle on your cancellation rate is the key.
Besides, mathematical formulae aside, it’s perhaps the single best and easiest measure of whether you’re actually delivering on your promises to customers.
And what’s more important than that?
This post was originally published at Blog.ASmartBear.com. Jason Cohen has shared this with Vistage to help members in the Software as a Service understand the implications of cancellation rates and how to utilize it as a metric in their businesses.
Category : Customer Engagement